2022
Conferences
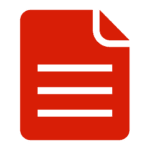
Igor Nascimento; Rinaldo Lima; Adrian Chifu; Bernard Espinasse; Sébastien Fournier
DeepREF: A Framework for Optimized Deep Learning-based Relation Classification Conference
Proceedings of the 13th Conference on Language Resources and Evaluation (LREC 2022), European Language Resources Association (ELRA), Marseille, France, 2022.
Abstract | Links | BibTeX | Tags: DDI, DeepREF, Embeddings, Framework, NLP, Optuna, Relation Classification, SemEval
@conference{ChifuLREC2022,
title = {DeepREF: A Framework for Optimized Deep Learning-based Relation Classification},
author = {Igor Nascimento and Rinaldo Lima and Adrian Chifu and Bernard Espinasse and Sébastien Fournier},
url = {http://www.lrec-conf.org/proceedings/lrec2022/pdf/2022.lrec-1.480.pdf},
year = {2022},
date = {2022-06-20},
urldate = {2022-06-20},
booktitle = {Proceedings of the 13th Conference on Language Resources and Evaluation (LREC 2022)},
pages = {4513–4522},
publisher = {European Language Resources Association (ELRA)},
address = {Marseille, France},
abstract = {Relation Extraction (RE) is an important basic Natural Language Processing (NLP) task for many applications, including search engines and question-answering systems. There are many studies in this subarea of NLP that continue to be explored, such as the ones concerned by SemEval shared tasks. For many years, several RE systems based on statistical models have been proposed, as well as the frameworks to develop them. We focus on frameworks allowing to develop such RE systems using deep learning models. Such frameworks make it possible to reproduce experiments using many deep learning models and preprocessing techniques. Currently, there are very few frameworks of this type. In this paper, we propose an open and optimizable framework called DeepREF, inspired by two other existing frameworks: OpenNRE and REflex. DeepREF allows the rapid development of deep learning models for Relation Classification (RC). In addition, it enables hyperparameter optimization, and the application of many preprocessing techniques on the input textual data. DeepREF provides means to boost the process of running deep learning models for RC tasks on different datasets and models. DeepREF is evaluated on three reference corpora and has demonstrated competitive results compared to other state-of-the-art RC systems.},
keywords = {DDI, DeepREF, Embeddings, Framework, NLP, Optuna, Relation Classification, SemEval},
pubstate = {published},
tppubtype = {conference}
}
2020
Conferences
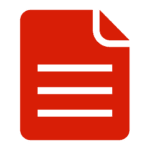
Francisco Rodrigues; Rinaldo Lima; William Domingues; Robson Fidalgo; Adrian Chifu; Bernard Espinasse; Sébastien Fournier
DeepNLPF: A Framework for Integrating Third Party NLP Tools Conference
Proceedings of the 12th Language Resources and Evaluation Conference, LREC2020 2020.
Abstract | Links | BibTeX | Tags: Framework, Natural Language Processing, NLP Tools Integration
@conference{rodrigues2020deepnlpf,
title = {DeepNLPF: A Framework for Integrating Third Party NLP Tools},
author = {Francisco Rodrigues and Rinaldo Lima and William Domingues and Robson Fidalgo and Adrian Chifu and Bernard Espinasse and Sébastien Fournier},
url = {http://www.lrec-conf.org/proceedings/lrec2020/pdf/2020.lrec-1.895.pdf},
year = {2020},
date = {2020-05-11},
urldate = {2020-01-01},
booktitle = {Proceedings of the 12th Language Resources and Evaluation Conference},
pages = {7244--7251},
series = {LREC2020},
abstract = {Natural Language Processing (NLP) of textual data is usually broken down into a sequence of several subtasks, where the output of one the subtasks becomes the input to the following one, which constitutes an NLP pipeline. Many third-party NLP tools are currently available, each performing distinct NLP subtasks. However, it is difficult to integrate several NLP toolkits into a pipeline due to many problems, including different input/output representations or formats, distinct programming languages, and tokenization issues. This paper presents DeepNLPF, a framework that enables easy integration of third-party NLP tools, allowing the user to preprocess natural language texts at lexical, syntactic, and semantic levels. The proposed framework also provides an API for complete pipeline customization including the definition of input/output formats, integration plugin management, transparent multiprocessing execution strategies, corpus-level statistics, and database persistence. Furthermore, the DeepNLPF user-friendly GUI allows its use even by a non-expert NLP user. We conducted runtime performance analysis showing that DeepNLPF not only easily integrates existent NLP toolkits but also reduces significant runtime processing compared to executing the same NLP pipeline in a sequential manner.},
keywords = {Framework, Natural Language Processing, NLP Tools Integration},
pubstate = {published},
tppubtype = {conference}
}