2018
Conferences
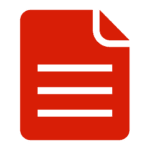
Adrian-Gabriel Chifu; Léa Laporte; Josiane Mothe; Md Zia Ullah
Query performance prediction focused on summarized letor features Conference
The 41st International ACM SIGIR Conference on Research & Development in Information Retrieval, SIGIR2018 2018.
Abstract | Links | BibTeX | Tags: Letor Features, Post Retrieval Features, Query Difficulty Prediction, Query Features, Query Performance Prediction
@conference{chifu2018query,
title = {Query performance prediction focused on summarized letor features},
author = {Adrian-Gabriel Chifu and Léa Laporte and Josiane Mothe and Md Zia Ullah},
url = {ftp://ftp.irit.fr/IRIT/SIG/2018_SIGIR_CLMU.pdf},
year = {2018},
date = {2018-07-01},
urldate = {2018-01-01},
booktitle = {The 41st International ACM SIGIR Conference on Research & Development in Information Retrieval},
pages = {1177--1180},
series = {SIGIR2018},
abstract = {Query performance prediction (QPP) aims at automatically estimating the information retrieval system effectiveness for any user's query. Previous work has investigated several types of pre- and post-retrieval query performance predictors; the latter has been shown to be more effective. In this paper we investigate the use of features that were initially defined for learning to rank in the task of QPP. While these features have been shown to be useful for learning to rank documents, they have never been studied as query performance predictors. We developed more than 350 variants of them based on summary functions. Conducting experiments on four TREC standard collections, we found that Letor-based features appear to be better QPP than predictors from the literature. Moreover, we show that combining the best Letor features outperforms the state of the art query performance predictors. This is the first study that considers such an amount and variety of Letor features for QPP and that demonstrates they are appropriate for this task.},
keywords = {Letor Features, Post Retrieval Features, Query Difficulty Prediction, Query Features, Query Performance Prediction},
pubstate = {published},
tppubtype = {conference}
}
2013
Conferences
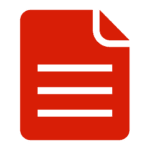
Adrian-Gabriel Chifu
Prédire la Difficulté des Requêtes: la Combinaison de Mesures Statistiques et Sémantiques Conference
COnférence francophone en Recherche d'Information et Applications, CORIA2013 2013.
Abstract | Links | BibTeX | Tags: Combined Predictors, Information Retrieval, Measure Correlation, Query Ambiguity, Query Difficulty, Query Performance Prediction
@conference{chifu2013predire,
title = {Prédire la Difficulté des Requêtes: la Combinaison de Mesures Statistiques et Sémantiques},
author = {Adrian-Gabriel Chifu},
url = {https://hal.archives-ouvertes.fr/hal-01145833/document},
year = {2013},
date = {2013-04-03},
urldate = {2013-01-01},
booktitle = {COnférence francophone en Recherche d'Information et Applications},
pages = {pp--191},
series = {CORIA2013},
abstract = {The performance of an Information Retrieval System (IRS) is closely related to the query. The queries that lead to retrieval failure are referenced in the literature as "difficult queries". This study aims at analysing, adapting and combining several difficulty predictors. The evaluation of the prediction is based on the correla- tion between the predicted difficulty and the IRS performance. As predictors, we have considered an ambiguity predictor, the IDF measure and a score distribution measure. We show that combining the proposed predictors, produce good results. The evaluation framework consists in the TREC7 and TREC8 ahdoc collections.},
keywords = {Combined Predictors, Information Retrieval, Measure Correlation, Query Ambiguity, Query Difficulty, Query Performance Prediction},
pubstate = {published},
tppubtype = {conference}
}