2022
Journal Articles
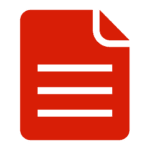
Ismail Badache; Adrian-Gabriel Chifu; Sébastien Fournier
Unsupervised and Supervised Methods to Estimate Temporal-Aware Contradictions in Online Course Reviews Journal Article
In: Mathematics, vol. 10, no. 5, 2022.
Abstract | Links | BibTeX | Tags: Aspect Detection, Contradiction Intensity, Feature Evaluation, Rating, Sentiment Analysis, Temporality
@article{badache2022,
title = {Unsupervised and Supervised Methods to Estimate Temporal-Aware Contradictions in Online Course Reviews},
author = {Ismail Badache and Adrian-Gabriel Chifu and Sébastien Fournier},
editor = {MDPI},
url = {https://www.mdpi.com/2227-7390/10/5/809},
doi = {10.3390/math10050809},
year = {2022},
date = {2022-03-03},
urldate = {2022-03-03},
journal = {Mathematics},
volume = {10},
number = {5},
abstract = {The analysis of user-generated content on the Internet has become increasingly popular for a wide variety of applications. One particular type of content is represented by the user reviews for programs, multimedia, products, and so on. Investigating the opinion contained by reviews may help in following the evolution of the reviewed items and thus in improving their quality. Detecting contradictory opinions in reviews is crucial when evaluating the quality of the respective resource. This article aims to estimate the contradiction intensity (strength) in the context of online courses (MOOC). This estimation was based on review ratings and on sentiment polarity in the comments, with respect to specific aspects, such as “lecturer”, “presentation”, etc. Between course sessions, users stop reviewing, and also, the course contents may evolve. Thus, the reviews are time dependent, and this is why they should be considered grouped by the course sessions. Having this in mind, the contribution of this paper is threefold: (a) defining the notion of subjective contradiction around specific aspects and then estimating its intensity based on sentiment polarity, review ratings, and temporality; (b) developing a dataset to evaluate the contradiction intensity measure, which was annotated based on a user study; (c) comparing our unsupervised method with supervised methods with automatic feature selection, over the dataset. The dataset collected from coursera.org is in English. It includes 2244 courses and 73,873 user-generated reviews of those courses.The results proved that the standard deviation of the ratings, the standard deviation of the polarities, and the number of reviews are suitable features for predicting the contradiction intensity classes. Among the supervised methods, the J48 decision trees algorithm yielded the best performance, compared to the naive Bayes model and the SVM model.},
keywords = {Aspect Detection, Contradiction Intensity, Feature Evaluation, Rating, Sentiment Analysis, Temporality},
pubstate = {published},
tppubtype = {article}
}
The analysis of user-generated content on the Internet has become increasingly popular for a wide variety of applications. One particular type of content is represented by the user reviews for programs, multimedia, products, and so on. Investigating the opinion contained by reviews may help in following the evolution of the reviewed items and thus in improving their quality. Detecting contradictory opinions in reviews is crucial when evaluating the quality of the respective resource. This article aims to estimate the contradiction intensity (strength) in the context of online courses (MOOC). This estimation was based on review ratings and on sentiment polarity in the comments, with respect to specific aspects, such as “lecturer”, “presentation”, etc. Between course sessions, users stop reviewing, and also, the course contents may evolve. Thus, the reviews are time dependent, and this is why they should be considered grouped by the course sessions. Having this in mind, the contribution of this paper is threefold: (a) defining the notion of subjective contradiction around specific aspects and then estimating its intensity based on sentiment polarity, review ratings, and temporality; (b) developing a dataset to evaluate the contradiction intensity measure, which was annotated based on a user study; (c) comparing our unsupervised method with supervised methods with automatic feature selection, over the dataset. The dataset collected from coursera.org is in English. It includes 2244 courses and 73,873 user-generated reviews of those courses.The results proved that the standard deviation of the ratings, the standard deviation of the polarities, and the number of reviews are suitable features for predicting the contradiction intensity classes. Among the supervised methods, the J48 decision trees algorithm yielded the best performance, compared to the naive Bayes model and the SVM model.
2018
Conferences
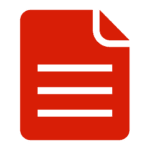
Ismail Badache; Sébastien Fournier; Adrian-Gabriel Chifu
Predicting Contradiction Intensity: Low, Strong or Very Strong? Conference
The 41st International ACM SIGIR Conference on Research & Development in Information Retrieval, SIGIR2018 2018.
Abstract | Links | BibTeX | Tags: Aspect, Contradiction Intensity, Feature Evaluation, Sentiment
@conference{badache2018predicting,
title = {Predicting Contradiction Intensity: Low, Strong or Very Strong?},
author = {Ismail Badache and Sébastien Fournier and Adrian-Gabriel Chifu},
url = {https://hal.archives-ouvertes.fr/hal-01796060/document},
year = {2018},
date = {2018-07-01},
urldate = {2018-01-01},
booktitle = {The 41st International ACM SIGIR Conference on Research & Development in Information Retrieval},
pages = {1125--1128},
series = {SIGIR2018},
abstract = {Reviews on web resources (e.g. courses, movies) become increasingly exploited in text analysis tasks (e.g. opinion detection, controversy detection). This paper investigates contradiction intensity in reviews exploiting different features such as variation of ratings and variation of polarities around specific entities (e.g. aspects, topics). Firstly, aspects are identified according to the distributions of the emotional terms in the vicinity of the most frequent nouns in the reviews collection. Secondly, the polarity of each review segment containing an aspect is estimated. Only resources containing these aspects with opposite polarities are considered. Finally, some features are evaluated, using feature selection algorithms, to determine their impact on the effectiveness of contradiction intensity detection. The selected features are used to learn some state-of-the-art learning approaches. The experiments are conducted on the Massive Open Online Courses data set containing 2244 courses and their 73,873 reviews, collected from coursera.org. Results showed that variation of ratings, variation of polarities, and reviews quantity are the best predictors of contradiction intensity. Also, J48 was the most effective learning approach for this type of classification.https://hal.archives-ouvertes.fr/hal-01796060/document},
keywords = {Aspect, Contradiction Intensity, Feature Evaluation, Sentiment},
pubstate = {published},
tppubtype = {conference}
}
Reviews on web resources (e.g. courses, movies) become increasingly exploited in text analysis tasks (e.g. opinion detection, controversy detection). This paper investigates contradiction intensity in reviews exploiting different features such as variation of ratings and variation of polarities around specific entities (e.g. aspects, topics). Firstly, aspects are identified according to the distributions of the emotional terms in the vicinity of the most frequent nouns in the reviews collection. Secondly, the polarity of each review segment containing an aspect is estimated. Only resources containing these aspects with opposite polarities are considered. Finally, some features are evaluated, using feature selection algorithms, to determine their impact on the effectiveness of contradiction intensity detection. The selected features are used to learn some state-of-the-art learning approaches. The experiments are conducted on the Massive Open Online Courses data set containing 2244 courses and their 73,873 reviews, collected from coursera.org. Results showed that variation of ratings, variation of polarities, and reviews quantity are the best predictors of contradiction intensity. Also, J48 was the most effective learning approach for this type of classification.https://hal.archives-ouvertes.fr/hal-01796060/document
TRANSLATE with
x
English
TRANSLATE with ![]()
Enable collaborative features and customize widget: Bing Webmaster Portal